Depth Thermal Conductivity Analysis by ML
Increasing heat generation density in semiconductor devices such as integrated circuits or power devices is an urgent issue. To evaluate thermophysical properties during device operation, it is desirable to obtain internal information in a non-contact and nondestructive manner.
FDTR is a method to estimate the thermal conductivity of a sample by periodically heating the sample surface with a pump light and measuring its temperature response with a probe light. It is expected to provide information on thermophysical properties that vary with depth, but currently there is a problem with how to process the data.
We are developing a method to analyze the depth-dependent thermal conductivity of a sample by applying machine learning to the FDTR. We generated frequency response data in the FDTR system, for various depth profiles of thermal conductivity, and trained them on a supervised machine learning model. We proposed a concise method for predicting thermal conductivity distribution in the depth direction from surface temperature information only.
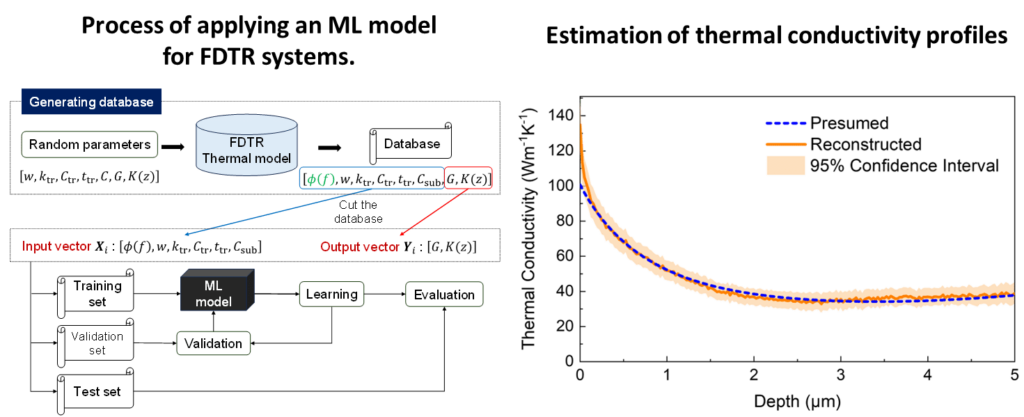