Physical Reservoir Computing with Heat Conduction
As the Internet of Things (IoT) continues to expand, reducing computational load has become increasingly important. One emerging approach to address this challenge is Physical Reservoir Computing (PRC), a machine learning model that exploits the transient state changes of nonlinear physical phenomena. PRC has gained attention for its potential to perform complex computations with low energy consumption and minimal processing overhead. In this study, we investigate the feasibility of PRC utilizing heat conduction phenomena, focusing on an alumina plate of a specific size as the computational medium. The heat conduction process exhibits dynamic and nonlinear behavior, making it a suitable candidate for reservoir computing. We evaluate the performance of our proposed PRC system through two benchmark tasks: the Short-Term Memory (STM) task and the Parity task. Our experimental results demonstrate that the PRC model based on heat conduction achieves consistent learning performance in both tasks. These findings indicate that heat conduction-based PRC is a promising alternative to conventional computing methods, potentially offering a more efficient and scalable approach for IoT applications. The ability to harness physical dynamics for machine learning tasks could lead to new computational paradigms with reduced energy consumption and enhanced processing efficiency.
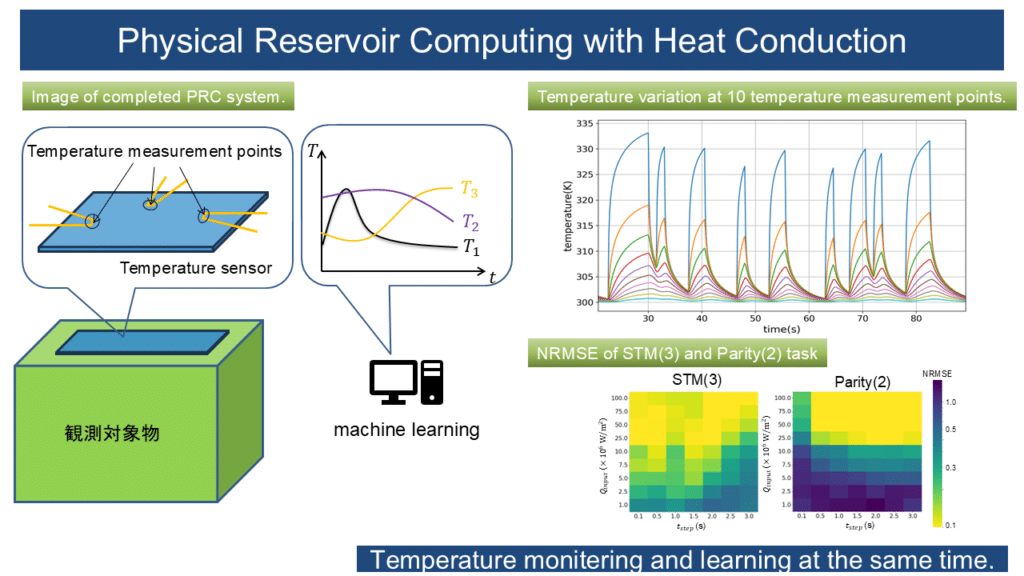